The Evolution of Fraud Detection: Part 1
- AM Fabi
- Jan 12, 2023
- 4 min read
Updated: Apr 3, 2023
From a mere 4% in 2007, India’s internet penetration rate saw an uptrend resulting in around 47% in 2021. That is nearly half of the population of 1.37 billion people had access to the internet that year, thus ranking the country second in the world in terms of active internet users. With such massive digital penetration in India, online banking has flourished tremendously in both rural and urban areas.
Due to the convenience that online transactions provide, consumers are increasingly choosing to use digital payments and online banking. As reported by RBI, the total digital payments increased from 3,40,026 lakh (2019-20) to 7,19,531 lakh (2021-22) in volume. However, safe crackers will always exist as long as there is a safe, as the saying goes. It is crucial that awareness is spread about such fraudsters and necessary actions are taken to curb their behavior as there has been a huge increase in financial scams where both consumers and banks are duped. The latest published report (as of 2020) by the National Crime Records Bureau (NCRB) indicates that the total number of cases registered under Fraud for cyber-crimes (including Credit/Debit Card, ATM, Online Banking Fraud, OTP Fraud & Others) have risen from 3466 (2017) to 10395 (2020) across Indian States & UTs.
Rule Based Systems for Fraud Detection
According to McKinsey, even in the current scenarios several financial organizations use rigidly codified rule- and scenario-based techniques for transaction monitoring. Such rules are majorly spurred by industry forewarnings, statistical indices, and professional expertise.
Rule based based systems, as the name suggests, rely on hard coded rules that are set to flag transactions if they meet certain criteria. Such rules can be developed by following the industry best practices - like blocking multiple transactions from a single account in a short period of time or the ones coming through VPNs or from risky areas, and then analyzing previously detected/caught fraudulent transactions leading to developing new rules to cover all of their suspicious characteristics.
In short, they mimic how a human would handle a transaction by checking to see if it fits any of the risky patterns specified in the rules and in case it does, block or forward it for manual human review – a huge reason why they are still ever present.
Banks have reacted fiercely to the rise in money laundering and other financial crimes, spending billions of dollars annually to strengthen their defenses against these crimes. LexisNexis Risk Solutions 2022 Real Cost of Financial Crime Compliance Research - Global Summary estimates that financial institutions worldwide will spend $274.1 billion on financial crime compliance in 2022, up from $213.9 billion in 2020. Also, when regulators impose harsher penalties, the resulting regulatory fines tied to compliance rise year over year. Yet, these conventional scenario- and rule-based methods to combating financial crimes have always looked to be one step behind the criminals, rendering the battle against money laundering a continual conundrum for compliance, monitoring, and risk organizations.
The Advent of Machine Learning in Fraud Detection
The continuous need of reverse engineering fraudsters’ attacks, incremental number of rules, limited complexity due to manual development of rules and necessary maintenance have paved the way for machine learning models to outshine rule based systems.
Machine learning models use more detailed, behavior-indicative data to develop multifaceted algorithms, making them more adaptable in swiftly catching up with changing trends and upgrading over time. Feedzai asserts that a well-tuned machine learning system can identify up to 95% of fraud and reduce the expense of human reconciliations, which now accounts for 25% of fraud expenditures. According to Capgemini, fraud detection systems which utilize machine learning and analytics significantly reduce the fraud investigation time by 70% and increase detection accuracy by 90%.
In contrast to the manual development of rules and the recalibration & adjusting to new fraud patterns, a collection of ML algorithms is trained with historical data to suggest risk rules which can then be implemented to block or allow certain user actions such as suspicious logins, identity theft, or fraudulent transactions.
When training the machine learning engine, previous cases of fraud and non-fraud must be flagged to avoid false positives and to improve the precision of your risk rules. The longer the algorithms run, the more accurate the rule suggestions will be.
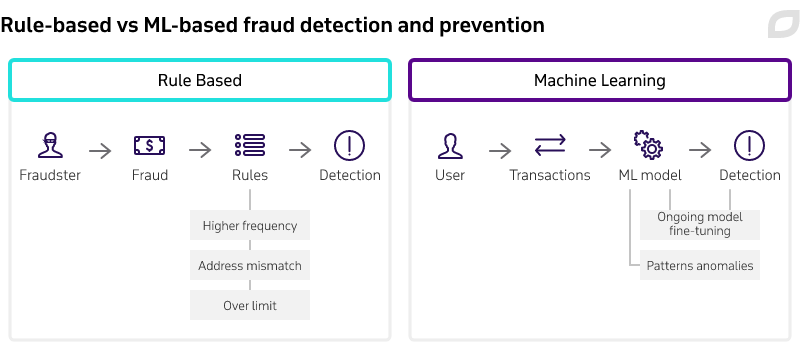
Since machines can swiftly process large datasets in comparison to us humans, the potential to slice and dice huge amounts of information is top-notch. This implies faster and efficient detection, reduced manual review time, better predictions with large datasets, cost-effective solution and many more perks.
For instance, a startup like Compliance.ai uses adaptive machine learning models in FinTech to automate research and track financial regulatory content and regulatory updates in a single platform. Also, companies like PayPal are using machine learning to enhance their fraud detection and risk management capabilities. Through a combination of linear, neural networks, and deep learning techniques, PayPal’s risk management engines can determine the risk levels associated with a customer within milliseconds.
It's not just banks or financial institutions that reap such benefits using ML. Such solutions are implemented to strengthen fraud detection in the insurance sector, especially in healthcare. Algorithms can easily identify false and duplicate claims like the case of a customer who reported an incorrect diagnosis or exaggerated medical coverage costs. Natural Language Processing techniques ensures in-depth analyses of unstructured data such as medical reports thereby, facilitating the scan of documents written by doctors, insurers, or clients, searching for suspicious inconsistencies.
Yet another example is tax fraud detection which can be done, for instance, by scrutinizing the general ledger in search of aberrant entries which might possibly be indicators of attempted fraud. By considering factors such as the monthly discrepancies in a company’s gross sales, taxpayer relationships, the fluctuations in purchases or itemized deductions in an income peer group, etc. algorithms can discern an exhaustive range of tip-offs quicker than your average human auditors.
Fraud attacks can happen 24*7, but even the best fraud managers may have to deal with a backlog of manual reviews. Machines can ease up the entire process by sorting through the obviously fraudulent or acceptable cases.
Automatic fraud pattern recognition, retraining on new data to often combat concept drift, economic efficiency are the notable advantages of adopting the machine learning ways, yet they pose certain challenges like extreme class imbalance, lack of full explainability of models’ predictions to name a few. Know more a overcoming these hurdles in the next part, so stay tuned!
Kommentarer